15th September
CET 17:00-18:15
Beijing 23:00-00:15
Sydney 01:00-02:15
New York 11:00-12:15
San Francisco 08:00-09:15
Time will Tell
Abstract: We are creatures of habit. This is reflected in our interactions with search engines and recommender systems. There is a a long line of work on understanding the time series underlying querying behavior and on understanding repeat consumption. Time and again, we find that temporal signals can help to improve search and recommendation effectiveness. The talk has two goals. One is to summarize recent advances in modeling and exploiting the temporal structure underlying people’s information needs in the setting of e-commerce. The other is to identify and prioritize gaps in our knowledge on the topic.
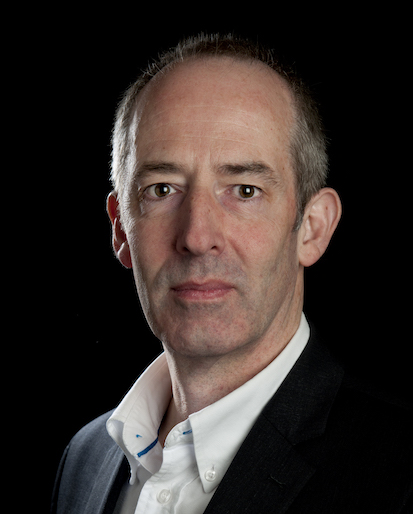
Maarten de Rijke
(Ahold Delhaize)
Maarten de Rijke is University Professor of Artificial Intelligence and Information Retrieval at the University of Amsterdam. He is also VP Personalization and Relevance and Senior Research Fellow at Ahold Delhaize. His research strives to build intelligent technology to connect people to information. His team pushes the frontiers of search engines, recommender systems and conversational assistants. They also investigate the influence of the technology they develop on society. De Rijke is the director of the Innovation Center for Artificial Intelligence.
Discovery in the Bloomberg Terminal
The Bloomberg Terminal delivers a diverse array of financial information, news and analytics to facilitate decision-making by professionals across the global capital markets. It encompasses hundreds of verticals like news, financial research, FX, equities and bonds, to name a few. The Terminal allows users to perform queries and run analyses over a wide array of structured and unstructured data. This wealth of functionality and data poses discovery challenges for new users or those who go beyond their usual workflows. Some examples of such discovery challenges include determining the right place to perform a specific analysis or determining the name of the correct field when crafting a query.
In this talk, I will present some of Bloomberg’s approaches to facilitating discovery, which lay at the intersection of information retrieval, natural language processing, and human-computer interaction. I will focus on two approaches: 1) natural language question answering and 2) semantic query auto-completion and suggestion. In addition to presenting some of the work done at Bloomberg in these two areas, I will present insights from this research that also apply to settings beyond Bloomberg, as well as some research problems we have come across that are of interest to the wider community.

Mohamed Yahya
(Bloomberg)
Mohamed Yahya is a researcher in the AI group at Bloomberg since 2016, where his work focuses on various aspects of discovery including semantic parsing for question answering, semantic auto-completion, aggregated auto-completion and other related problems.
His other research interests include information extraction, knowledge graphs, and combining structured and unstructured data for question answering. Mohamed’s research lies at the intersection of the IR, NLP, and database communities, with publications appearing at EMNLP, NAACL, WWW, WSDM, SIGIR, and VLDB, among others. Before joining Bloomberg, Mohamed earned his doctoral degree from the Max Planck Institute for Informatics and interned with Google Research and Siemens Corporate Research and Technology.